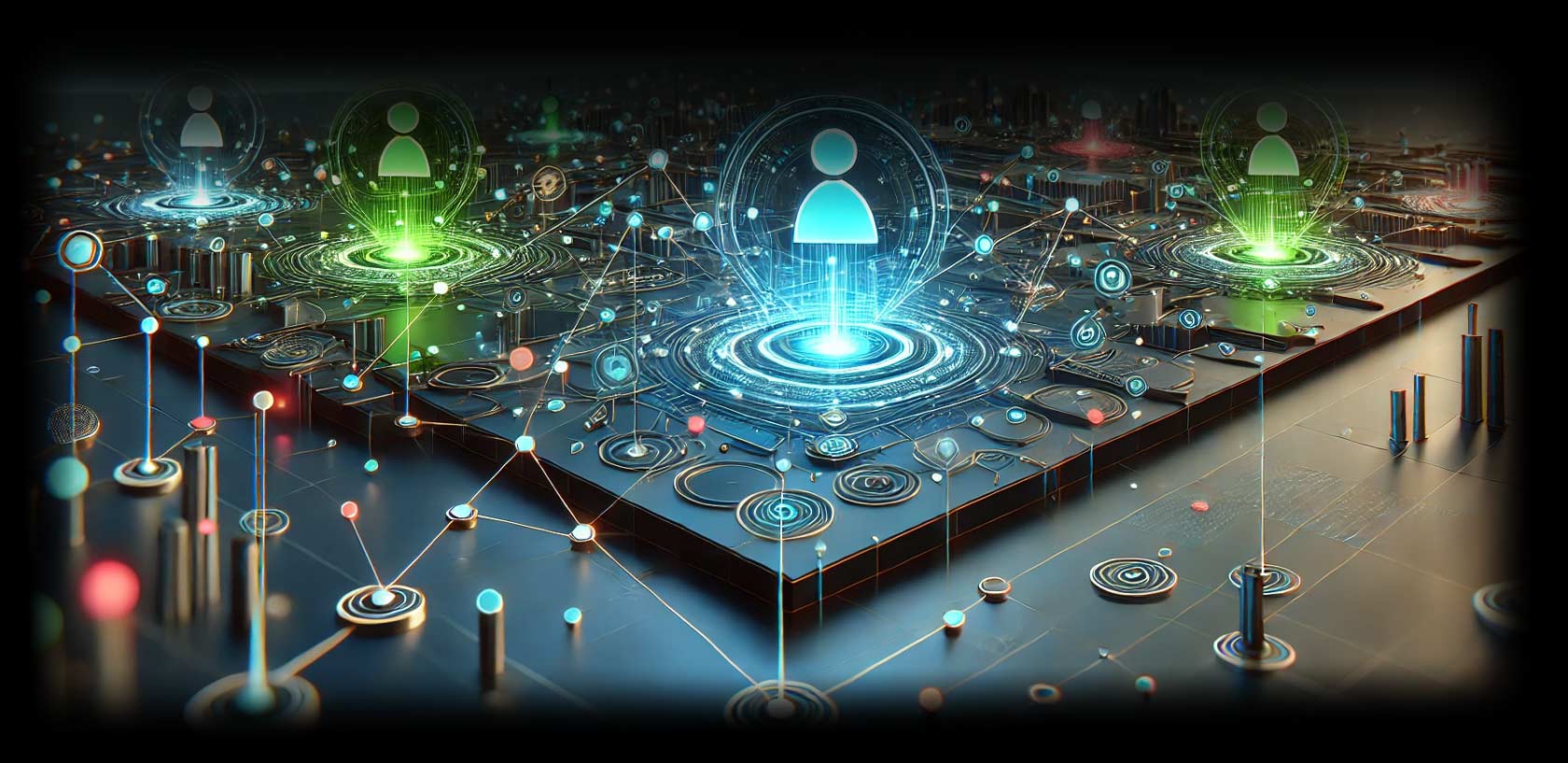
AI agents at a glance: AI single, multi-, sequential and swarm agents for modern business processes
A comprehensive guide to the different types of agents in the field of artificial intelligence and their application in marketing, knowledge management, customer support, content creation and software development.
In today’s digital era, artificial intelligence (AI) is playing an increasingly important role in our daily lives and in business processes worldwide. From personalized recommendations in online stores to complex automation systems in industry – the applications are diverse and revolutionary. One particularly exciting field within AI is the development and use of AI agents. These intelligent systems are designed to perform tasks independently, make decisions and interact with their environment.
This page aims to give you a comprehensive overview of the different types of AI agents, in particular AI Single Agents, Multi Agents, Sequential Agents and Swarm Agents. We will explain basic concepts, present specific examples and show how these agents are used in different application areas. In addition, we would like to offer you the opportunity to experience these agents interactively by offering you a free demo where you can try them out directly.
The advantages of AI agents
Automated processes & relief
AI agents take over repetitive tasks, relieve your team and allow you to focus on value-adding activities.
Faster decisions & efficiency
AI-supported analysis provides you with relevant information in real time for better and faster decisions.
24/7 support & scalability
Whether customer service or internal processes - AI agents are available at all times and scale flexibly with your growth.
Types of AI agents
Single AI Agents
Individual AI agents operate independently of each other and are designed for specific tasks or use cases. They are specialized to perform a specific function efficiently and effectively without interacting with other agents.
Research AI Agent Pro
The Research AI Agent Pro specializes in efficiently collecting, analysing and providing information in a targeted manner. By searching through complex data sets in a targeted manner, it can extract relevant findings and provide precise answers to a wide range of questions. He researches current events, analyzes scientific articles and compares different sources to provide well-founded insights.
Its aim is to serve as a reliable source of knowledge, support sound decision-making processes, solve problems efficiently and impart knowledge in a targeted manner.
Areas of application:
✅ Automated market research – analysis of industry trends, customer behavior and competitive strategies to optimize business decisions.
✅ Scientific analysis – Systematic evaluation and comparison of studies, specialist articles and research results for scientists and experts.
✅ Competition monitoring – Continuous monitoring and analysis of market changes, competitor strategies and innovation developments.
AI Knowledge-Management Agent
The AI Knowledge Management Agent revolutionizes corporate knowledge management by structuring, intelligently organizing and providing information in a targeted manner. It only captures, categorizes and analyses internal company knowledge and is not connected to external online sources. This keeps the focus on company-relevant information and avoids distractions caused by general web queries – such as the weather or the latest news.
With its ability to quickly identify and provide relevant information, it not only optimizes internal communication, but also increases productivity and decision-making quality within the company. It helps teams to manage their knowledge centrally, avoid duplication of work and speed up access to company-relevant information.
Areas of application:
✅ Efficient knowledge management – automatic capture, structuring and provision of corporate knowledge for seamless information processing.
✅Internal communication & employee support – providing relevant information for teams to optimize processes and work more productively.
✅ AI-supported decision-making – analysis and provision of strategically important information to support data-based decisions.
With the AI Knowledge Management Agent, knowledge is not only collected, but actively harnessed to make companies smarter, more efficient and more competitive – without the distraction of external queries.
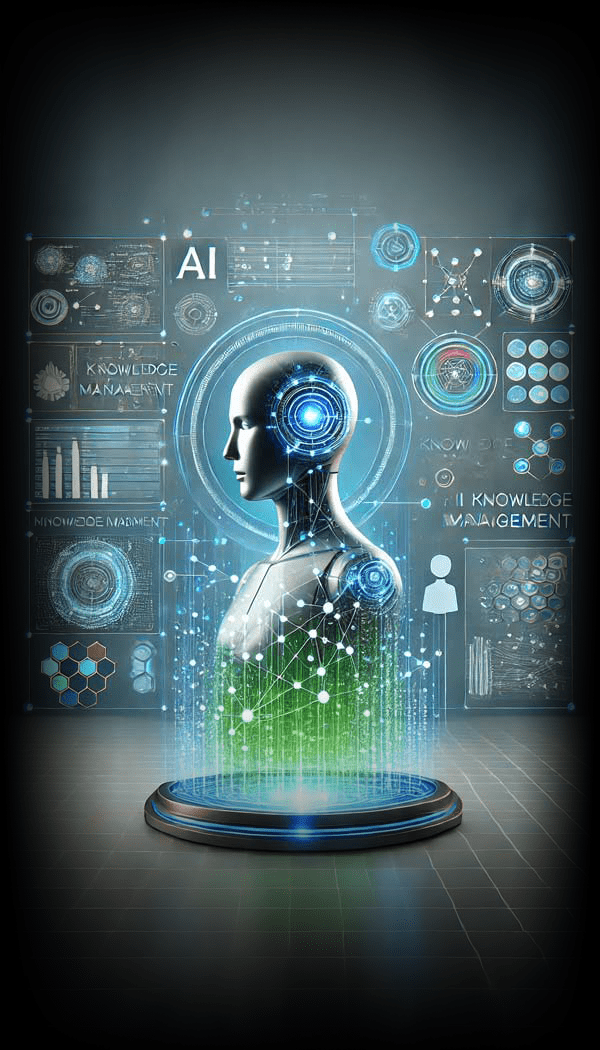
AI Multi Agents
Multi-agents consist of several networked AI agents that work together cooperatively and competitively to solve complex tasks. These agents can exchange information, divide up tasks, develop an overall goal from individually set objectives and make joint decisions, which leads to greater efficiency and flexibility.
Advantages of Multi Agents
Scalability: Several agents can work in parallel, which increases the processing capacity.
Robustness: If one agent fails, others can take over the tasks, making the system more resilient. This is particularly useful in critical systems, such as power grids (smart grids), where redundant agents are used.
Flexibility: Different agents can specialize in different aspects of a task, enabling a more comprehensive solution.
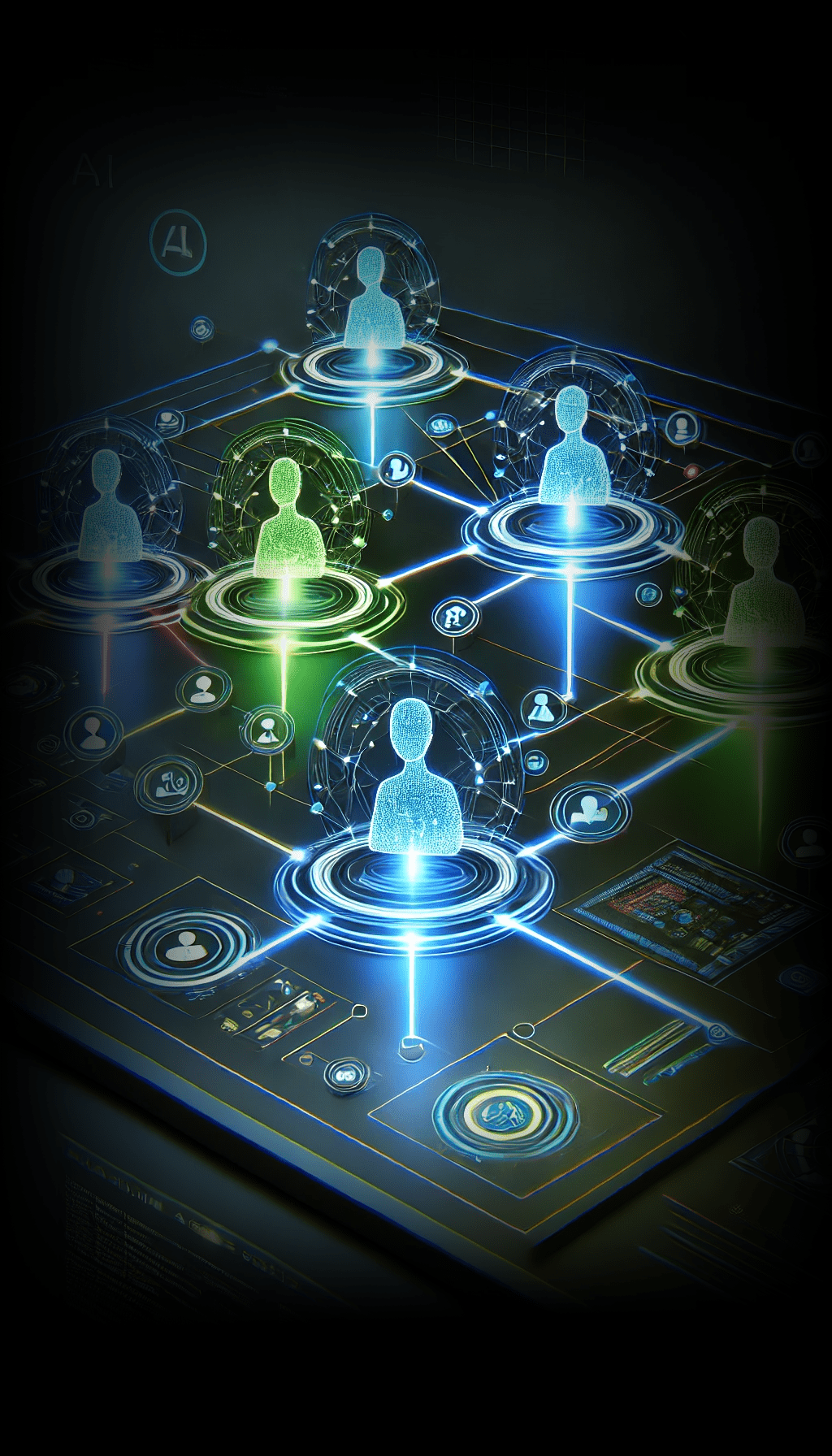
Content Creator AI Multi Agent Team
The Content Creator AI Multi-Agent Team is an intelligent system of specialized AI agents that automates and optimizes creative processes. By combining different agents, it can research and structure topics and generate high-quality content that is precisely tailored to the respective target group and platform.
Each agent takes on a specific task in the creative workflow. The interaction of these agents ensures efficient, consistent and high-quality content production that can be used for blogs, social media, whitepapers and marketing campaigns. As the agent team revises and improves the text several times, there may be several iterations – the user should therefore wait until the final optimized content is ready.
Functions and advantages:
✅ AI-supported text creation – generation of high-quality content that meets the requirements of different formats and platforms.
✅ Collaborative agent architecture – Specialized AI agents work together to manage the entire creative process.
✅Dynamic adaptation to content needs – Flexible scaling and optimization according to content requirements and business goals.
The Content Creator AI Multi-Agent Team enables organizations to produce high-quality content faster and more efficiently, while ensuring the consistency and relevance of the content created. The iterative optimization process ensures that content is revised multiple times to achieve the best possible quality.
Software Development AI Multi Agent Team
The Software Development AI Multi-Agent Team optimizes the entire software development process through specialized AI agents. They take care of coding, testing and documentation and work together collaboratively to maximize efficiency and quality.
Agents & Functions:
✅ Developer Agent – Creates clean, optimized code according to best practices.
✅ Reviewer Agent – Checks code for errors, security vulnerabilities and optimization potential.
✅ Documentation Agent – Creates technical documentation.
This intelligent division of labour makes the development process faster, safer and more structured, while minimizing errors and optimizing workflows.
Sequential Agents
Sequential agents work in a predefined sequence of steps or actions to achieve a specific goal. They are particularly useful in processes that require a clear sequence of tasks, such as the management of complex workflows.
Advantages of sequential agents
What makes sequential agents particularly powerful, however, is their ability to run through individual steps several times if additional iterations are required. This flexibility enables them to optimize sub-steps, check intermediate results and continuously improve. They can also communicate repeatedly with various other agents within a team to exchange information or coordinate specific tasks. These repeated interactions and feedback not only ensure a high level of efficiency, but also exceptional precision in achieving complex goals.
Content Flow Agent
The Content Flow Agent is a sequential agent that automates the entire content creation process. From keyword and topic research, writing and editing to publishing and distributing the content, it ensures a seamless and efficient workflow.
Workflow Coordinator Agent
The Workflow Coordinator Agent is a sequential agent team adapted to specific workflows that is used in companies to automate business processes. It ensures that all steps of a workflow are executed in the correct order and iteratively.
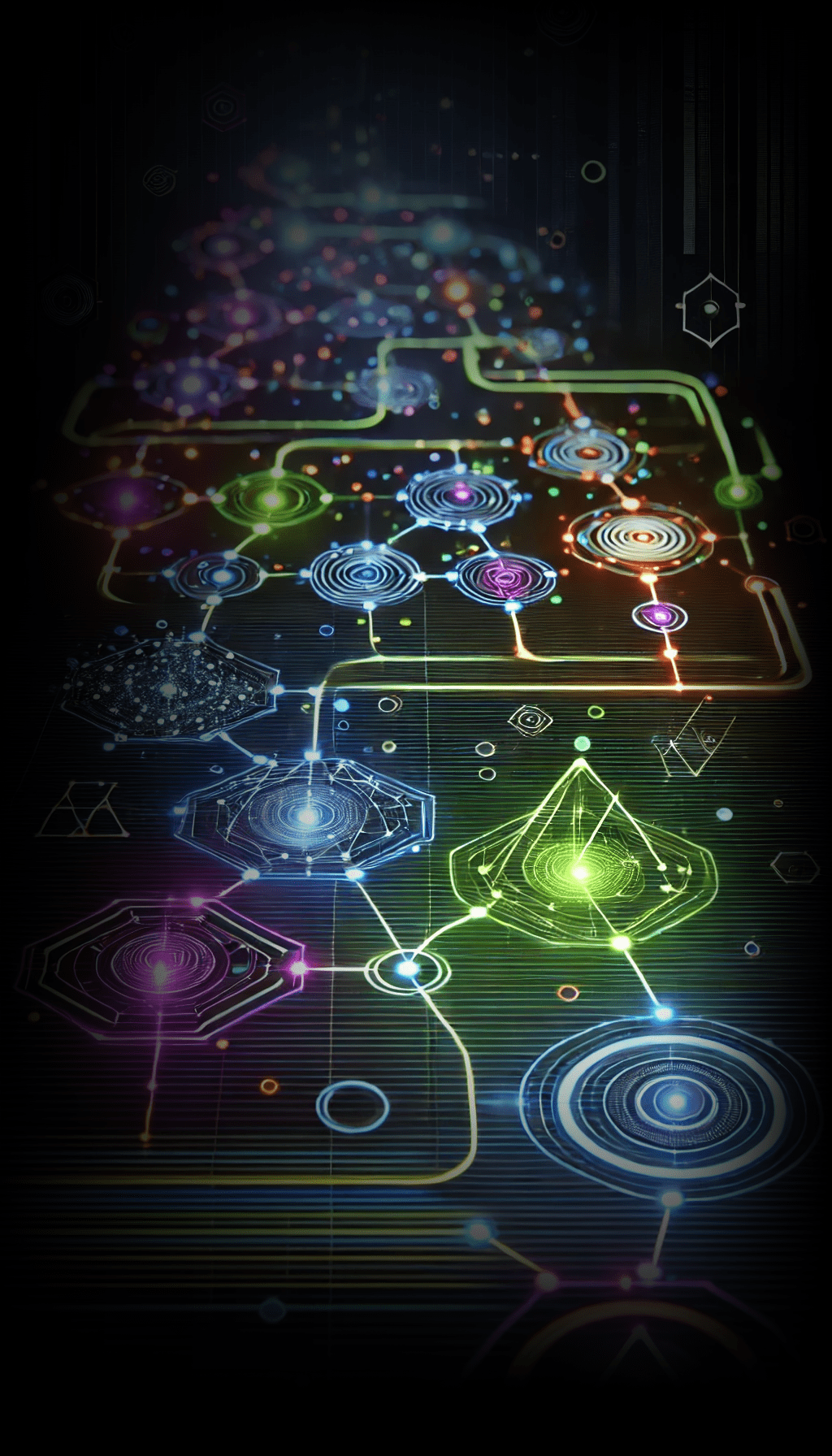
Swarm Agents
Swarm agents are inspired by natural swarm behavior. A swarm agent system consists of many cooperating agents that work decentrally without central control. These agents use local interactions and simple rules to accomplish complex tasks together. The collective intelligence of swarm agents makes it possible to adapt dynamically to changes and offer scalable solutions.
Advantages of Swarm Agents
Decentralized control: No single point of failure, which increases robustness and reliability.
Scalability: Easy addition or removal of agents without major impact on the overall system.
Adaptability: Ability to adapt quickly to changing conditions and requirements.
Flexibility: Suitable for a variety of tasks, from optimization to monitoring and data collection.
Project Swarm Agent
The Project Swarm Agent is a swarm agent system used in collaborative project work. The agents coordinate independently to distribute tasks, monitor progress and ensure that all project goals are achieved. This promotes efficient and effective collaboration in teams.
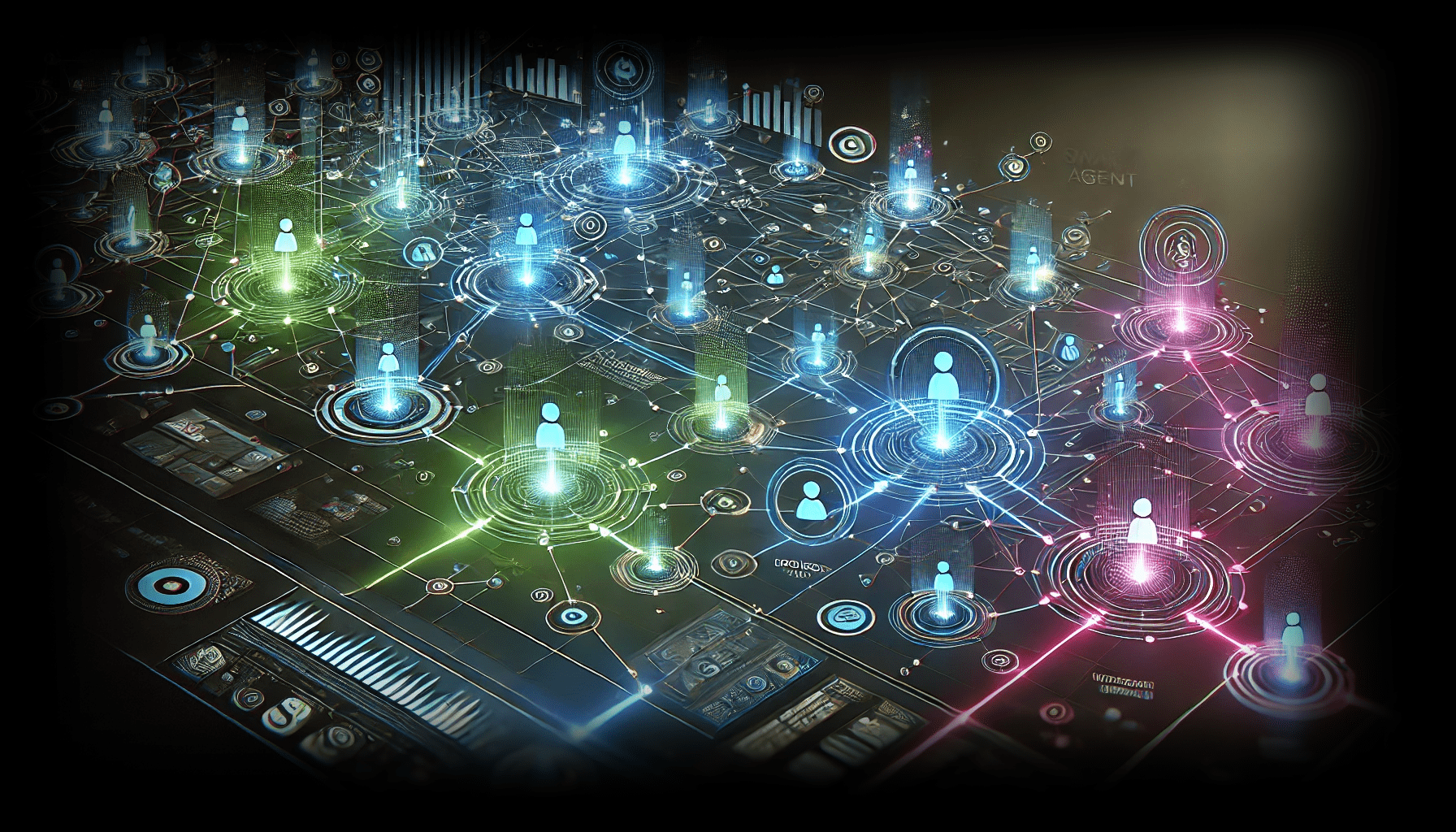
Interaction and communication
Interaction and communication between AI agents and their users and with each other is an essential part of how they work.
User interaction
AI agents use various interfaces such as chatbots, voice assistants or graphical user interfaces to interact with users. They understand requests, provide answers and perform tasks based on user input.
Agent interaction
In multi-agent systems, the individual agents communicate with each other to exchange information, coordinate tasks and make joint decisions. In swarm agent systems, communication is decentralized and often based on simple rules and local interactions.
Data integration
AI agents integrate data from various sources to gain comprehensive insights and make informed decisions. This can include the integration of real-time data, historical data or external data sources.
Areas of application and use cases
Diverse areas of application and innovative uses: How AI agents are enriching our everyday lives and revolutionizing business processes.
Business-oriented applications
AI agents in the business environment: AI agents are increasingly being used in areas such as resource optimization, monitoring and dynamic adaptation to market changes. For example, AI agents can be used in marketing to react to market trends in real time and dynamically adapt campaigns, or in knowledge management to efficiently collect and provide information.
Marketing: Multi-agent systems coordinate different marketing channels and optimize campaigns through the collaboration of specialized agents for SEO, social media and email marketing.
Content creation: Sequential agents automate the entire content creation process, from topic research and writing to publishing and distribution, improving efficiency and consistency.
Knowledge management: AI agents support the systematic collection, categorization and provision of knowledge within a company, which improves information accessibility and decision-making.
Customer support: Multi-agent systems in customer support enable efficient processing of inquiries by specialized agents who cover various support channels such as chat, email and telephone.
Software development: In software development, multi-agents can be used to process different modules or components of a project at the same time, while sequential agents divide the development process into phases such as planning, coding, testing and deployment.
Economy and industry
AI agents are used in a variety of ways in business and industry to optimize processes and increase efficiency.
Supply chain management: AI agents monitor and optimize supply chains by managing inventories, predicting delivery times and preventing bottlenecks.
Financial analysis: In the financial sector, AI agents analyze market data, create forecasts and support investment decisions.
Production automation: In manufacturing, AI agents control production lines, monitor quality controls and optimize the use of resources.
Education and research
In education and research, AI agents offer innovative opportunities for knowledge transfer and scientific discovery.
Intelligent tutors: AI agents support pupils and students with personalized learning plans, feedback and interactive exercises.
Research assistance: In research, AI agents analyze large data sets, identify relevant information and support the development of hypotheses.
Educational administration: AI agents optimize administrative processes in educational institutions, such as the administration of course offerings and the analysis of performance data.
Healthcare
In the healthcare sector, AI agents are helping to improve patient care and the management of healthcare facilities.
Diagnostic support: AI agents analyze medical images and data to help doctors diagnose diseases.
Personalized medicine: By analyzing genetic and clinical data, AI agents support the development of individual treatment plans.
Administrative tasks: AI agents automate administrative tasks such as appointment scheduling, billing and patient management, freeing up medical staff.
Other areas of application
In addition to the areas mentioned, AI agents are also used in many other fields.
Security: AI agents monitor security cameras, detect anomalies and help prevent security incidents.
E-commerce: Personalized recommendation systems analyse user behaviour and suggest products that match their preferences.
Logistics: AI agents optimize route planning, warehouse management and delivery processes to increase efficiency and customer satisfaction.
Entertainment: In the gaming industry, AI agents create realistic opponents and allies that enrich the gaming experience.
FAQ
Frequently asked questions
A single AI agent operates independently and specializes in a specific task. A multi-agent system consists of several agents that interact and cooperate with each other in order to handle more complex tasks and achieve greater efficiency.
Multi Agents, Sequential Agents and Swarm Agents differ fundamentally in their structure and functionality, especially with regard to their application in business processes:
Multi Agents:
These systems consist of multiple, often specialized agents that work in parallel or cooperatively to accomplish complex tasks. Unlike sequential agents, multi-agents can communicate and interact with each other freely and dynamically in all directions. In business areas such as marketing and customer support, multi-agents enable tasks to be processed more efficiently and flexibly.
For example, a multi-agent system in marketing can deploy different agents who are each responsible for social media management, email campaigns and market analyses. Each agent can not only act independently, but can also exchange data and insights with all other agents in real time and obtain feedback in order to continuously optimize strategies. This dynamic collaboration makes it possible to develop and flexibly adapt holistic marketing strategies.
In customer support, different agents can simultaneously process inquiries, set priorities and manage escalations. Through the constant exchange of information and best practices between agents, solutions are continuously improved, response times are shortened and customer satisfaction is increased. Agents can support each other, share experiences and, if necessary, redistribute tasks to achieve optimal results.
Sequential agents:
These agents work primarily in a set sequence of actions, which is particularly useful for processes that require a clearly defined sequence. It is important to understand that the process not only moves forward in a linear fashion, but also allows for iterative feedback.
In content creation, for example, sequential agents can run through the phases of content creation – from topic research and writing to publication and distribution. Each step is handled by an agent, who not only passes the output on to the next agent, but can also send it back to previous agents for revision if necessary.
This enables continuous optimization and quality improvement. In knowledge management, sequential agents can be used to systematically collect, categorize and provide information, with each agent taking on a specific task in data processing and contributing to the refinement of results through feedback loops.
Swarm agents:
In the business environment, swarm agents find application in areas such as optimization of resources, monitoring and dynamic adaptation to market changes. For example, swarm agents can be used in marketing to react to market trends in real time and dynamically adapt campaigns, or in knowledge management to efficiently collect and provide information. Swarm agents work decentrally and use local interactions to achieve global goals, which is particularly advantageous in dynamic and distributed task areas.
Example:
In software development, multi-agents can be used to work on different modules or components of a project at the same time, while sequential agents divide the development process into phases such as planning, coding, testing and deployment. Swarm agents, on the other hand, can be used to optimize resource allocation and dynamically adapt to changing requirements.
All three agent types offer advantages in terms of efficiency and automation, with multi-agents being particularly effective in dynamic and distributed task areas, sequential agents in structured and linear processes and swarm agents in decentralized and adaptable task areas.
Swarm agents are inspired by natural swarm behavior and consist of many simple but cooperating agents that work in a decentralized manner. They use local interactions and simple rules to tackle complex tasks together without centralized control. In comparison:
- Multi-agents consist of several specialized agents that work together cooperatively or competitively to solve complex tasks, often with some centralization or coordination.
- Sequential agents work in a fixed sequence of steps or actions to achieve a specific goal, which is particularly useful in structured processes.
Use in business processes:
- Marketing: Swarm agents can react to market changes in real time, dynamically adapt campaigns and efficiently distribute resources. For example, they can optimize different marketing channels simultaneously by analyzing local data and making decisions to improve the overall strategy.
- Content creation: Swarm Agents can work together to research, write, edit and publish content. Each agent takes on a specific sub-process and shares the results with the others to ensure a seamless and efficient workflow.
- Knowledge management: Swarm agents can collect, categorize and provide information from various sources by working in a decentralized manner and using local interactions. This enables efficient and comprehensive management of corporate knowledge.
- Customer Support: Swarm Agents can simultaneously handle, prioritize and escalate multiple customer requests across multiple channels, reducing response times and increasing customer satisfaction.
- Software development: Swarm agents can optimize resource allocation by working on different parts of a software project in parallel and supporting each other to improve the efficiency and quality of development.
Swarm Agents offer a flexible and robust solution for complex and dynamic business processes thanks to their decentralized and collaborative way of working.
The safety of autonomous AI agents depends on various factors, including the quality of the algorithms, the data on which they have been trained and the implementation of safety protocols. It is important to implement strict safety standards and regular reviews to minimize potential risks.
To implement an AI agent, you need access to relevant data, a suitable infrastructure for training and running the agents and expertise in machine learning, programming and data management. In addition, clear objectives and careful planning are required to successfully integrate the agent into existing systems.
AI agents can automate certain tasks and thus supplement human labor. While some repetitive and standardized jobs could be replaced by AI agents, new fields of work and requirements for qualified specialists who are able to work with and monitor AI technologies are emerging at the same time.
AI agents learn in three main ways:
- Supervised learning:
- The agent receives examples with correct solutions
- Like a student practicing tasks with sample solutions
- Example: Recognizing spam e-mails using marked examples
- Unsupervised learning:
- The agent independently searches for patterns in data
- Like a child who discovers similarities on its own
- Example: Sort customer groups according to purchasing behavior
- Reinforcement learning:
- The agent learns through trial and error
- Like a dog that is rewarded for correct behavior
- Example: AI learns computer games through success and failure
All these methods are based on the fact that the agent learns from large amounts of data and continuously improves its performance.
Multi-agent systems offer advantages such as greater scalability, robustness and flexibility. More complex tasks can be solved more efficiently by several agents working together, and the system is more resistant to failures of individual agents.
Sequential agents are often used in content creation, knowledge management, software development and business processes such as marketing and customer support. They are ideal for tasks that require a clear sequence of steps, such as the automation of content creation processes, the systematic collection and provision of knowledge or the structuring of software development cycles.
Autonomous AI agents can make decisions on their own and adapt to changing environments, while traditional software programs execute predefined instructions without the ability to self-adapt or make decisions. Autonomous agents use machine learning and other AI techniques to continuously improve their performance.
When using AI agents, ethical considerations such as data protection, transparency, accountability, fairness and the avoidance of bias in the algorithms must be taken into account. It is important to develop and adhere to ethical guidelines to ensure that AI agents are used responsibly and for the benefit of society.
Yes, AI agents can communicate with each other in multi-agent systems. This is done through defined communication protocols and APIs that enable the exchange of information, coordination of tasks and joint decision-making. In swarm agent systems, communication is decentralized and often based on simple rules and local interactions. Communication can be text-based, verbal or via special data formats.
AI agents are based on various technologies such as machine learning, deep learning, natural language processing (NLP), computer vision, reinforcement learning and neural networks. These technologies enable the agents to learn from data, understand natural language, process visual information and make autonomous decisions.
Companies can achieve the successful use of AI agents by setting clear objectives, selecting suitable use cases, ensuring data quality, integrating with existing systems and training employees. It is also important to develop ethical guidelines and continuously monitor the performance and safety of the agents.
Reactive AI agents:
- Acting as a reflex to current situations
- React only to the “here and now”
- Example: A thermostat that only reacts to current temperature changes
Proactive AI agents:
- Think ahead and plan
- Can anticipate and prepare for future situations
- Example: A smart home system that switches on the heating before you get home
The main difference is that reactive agents react like an autopilot to immediate stimuli, while proactive agents act like a forward-looking navigator that plans the route and recognizes potential problems early on.
AI agents analyse individual user data and behaviour patterns in order to offer personalized recommendations and tailored services. This increases user satisfaction and can strengthen customer loyalty by better tailoring the solutions offered to users‘ needs and preferences.
AI agents will play a central role in the automation of tasks that are repetitive or data-intensive. They will complement human workers by taking over routine tasks and giving employees more time for creative and strategic activities. They will also create new fields of work that require specialized skills in AI and data analysis.
Advantages and challenges of AI agents
Advantages
The use of AI agents offers numerous advantages that benefit both companies and individuals:
- Increased efficiency: AI agents automate repetitive tasks, resulting in significant time and cost savings.
- Improved decision-making: By analyzing large amounts of data, AI agents provide in-depth insights that support decision-making.
- Personalization: AI agents enable customized solutions and services that are tailored to the individual needs and preferences of users.
- Scalability: AI agents can be easily scaled to handle increasing demands and volumes.
- Continuous learning: AI agents continuously improve through machine learning, leading to ever better performance.
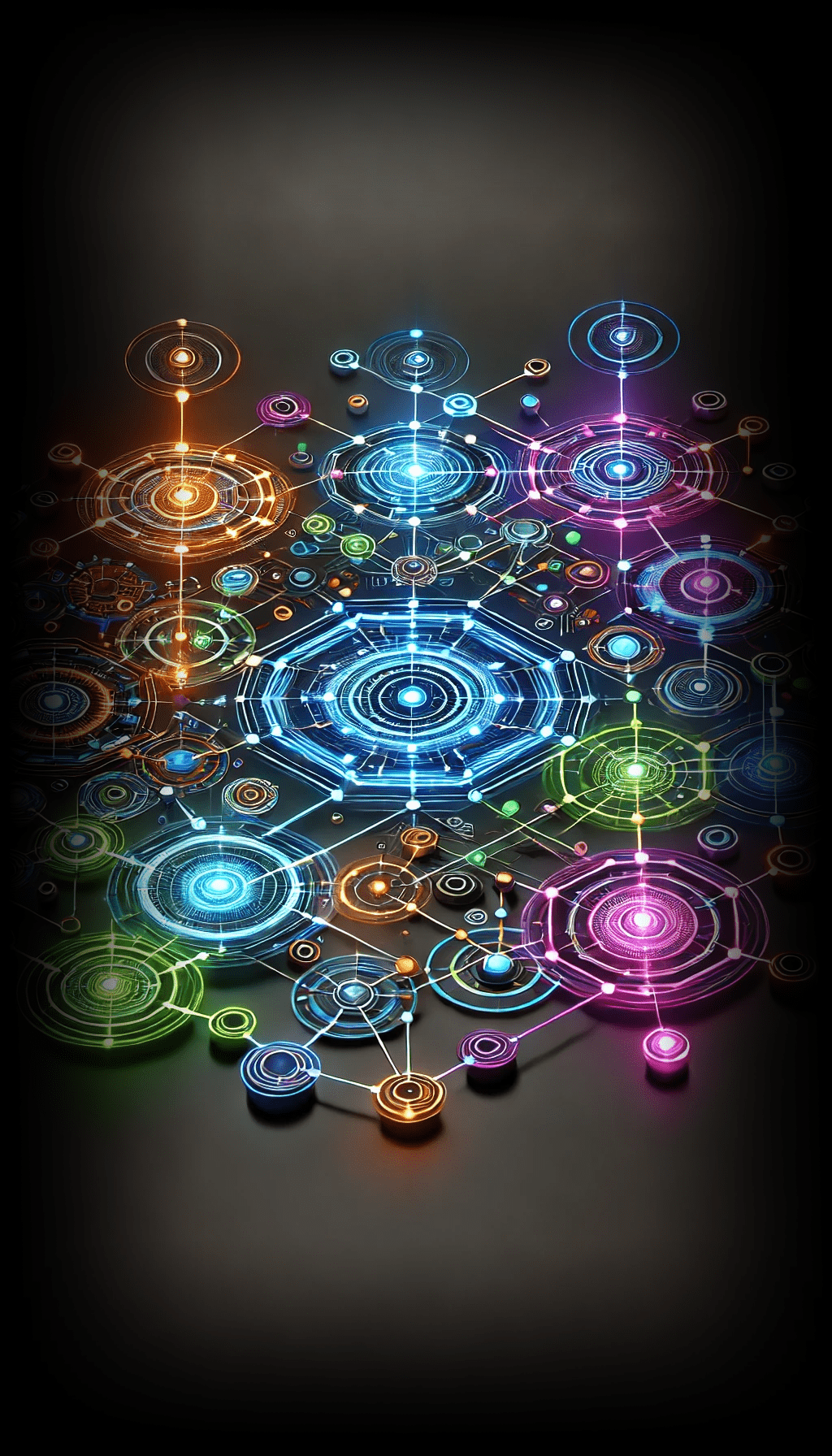
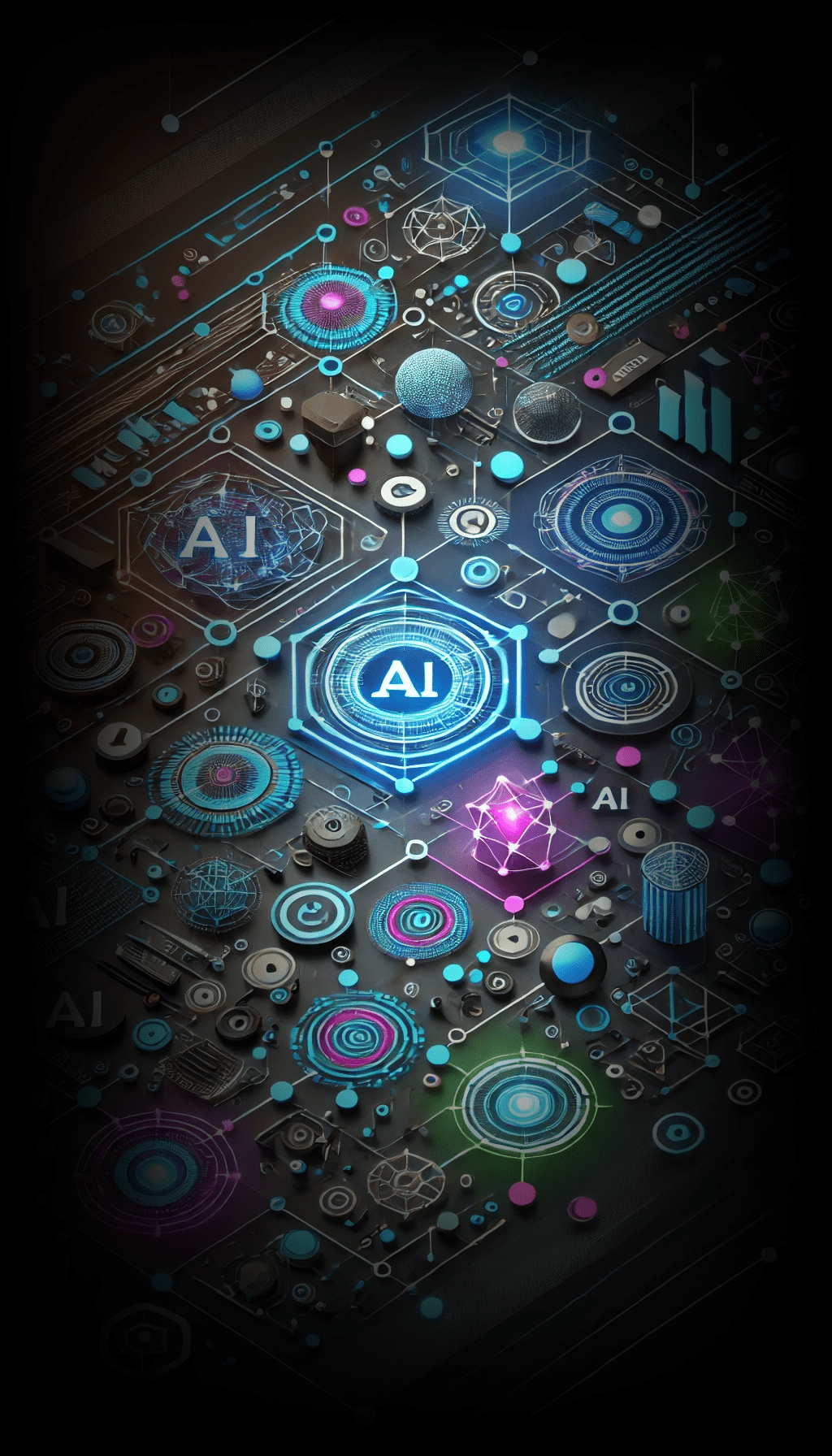
Challenges
- Data protection: The handling of sensitive data requires strict security measures and compliance with legal regulations in order to protect the privacy of users.
- Ethical issues: The use of AI agents raises ethical issues, such as responsibility for decisions made by agents and the avoidance of bias in the algorithms.
- Complexity of implementation: Integrating AI agents into existing systems can be technically complex and resource-intensive.
- Transparency: Many AI models, especially deep learning algorithms, are often known as “black boxes”, which makes it difficult to understand and make decision-making processes transparent.
- Workplace changes: Automation through AI agents can lead to changes in the workplace, including the need for new skills and potential job losses in certain areas.
Future developments
The future of AI agents promises exciting developments and innovations:
- Improved interactivity: AI agents are getting better at understanding and using natural language, making interaction with them more natural and intuitive.
- Increased autonomy: Future AI agents will be even more autonomous and able to handle more complex tasks without human intervention.
- Integration of multimodality: The ability to process different data sources and types (e.g. text, image, audio) simultaneously will further increase the performance of AI agents.
- Ethical AI: Advances in ethical AI research will help to develop fairer and more transparent AI agents.
- Interdisciplinary applications: AI agents will increasingly be used in interdisciplinary fields, creating innovative solutions to complex global challenges.
- Business-specific applications: The development of specialized Multi Agents, Sequential Agents and Swarm Agents for specific business processes in areas such as marketing, content creation, knowledge management, customer support and software development will continue to grow to increase efficiency and effectiveness in these areas.
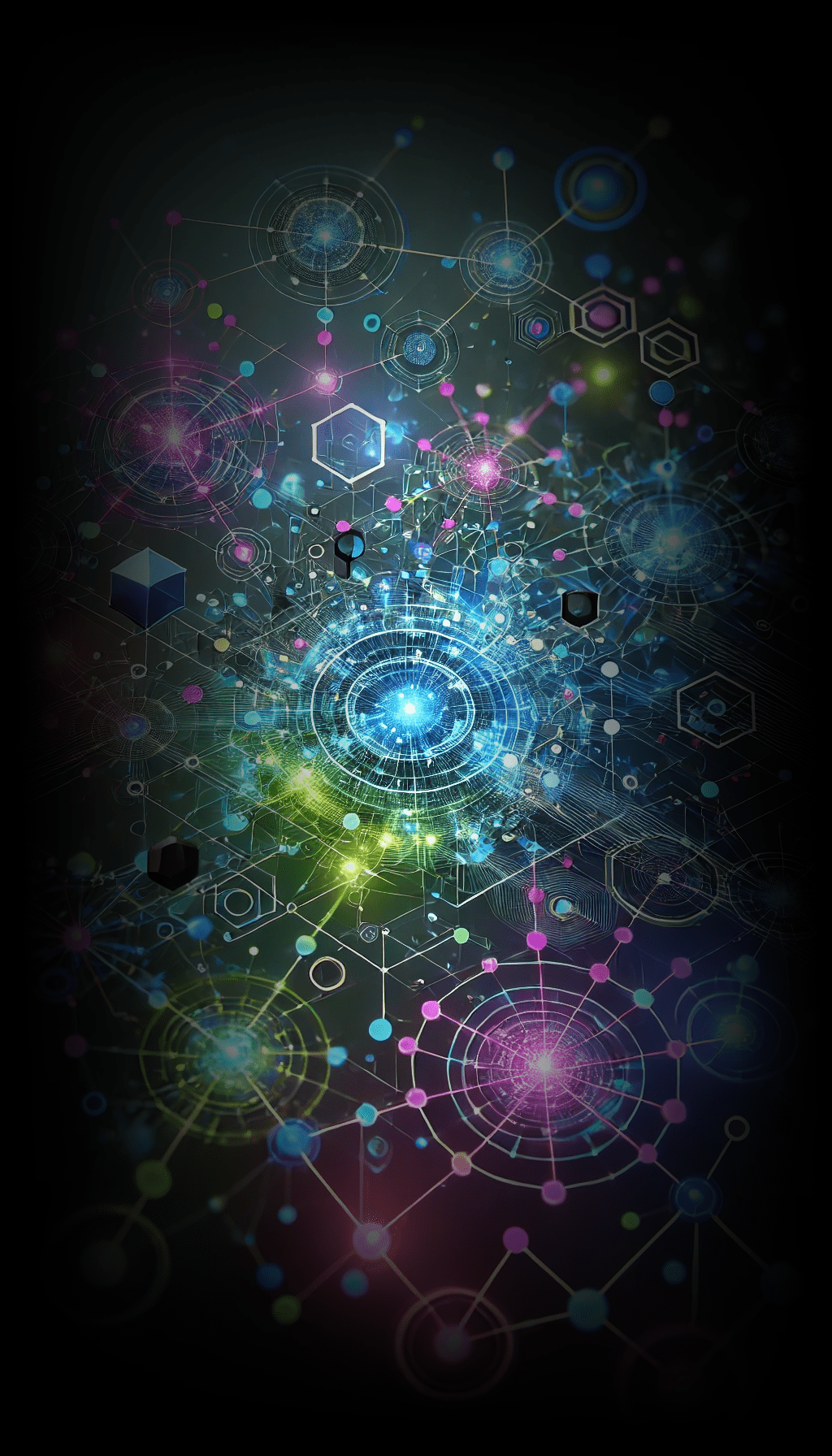
Conclusion and outlook
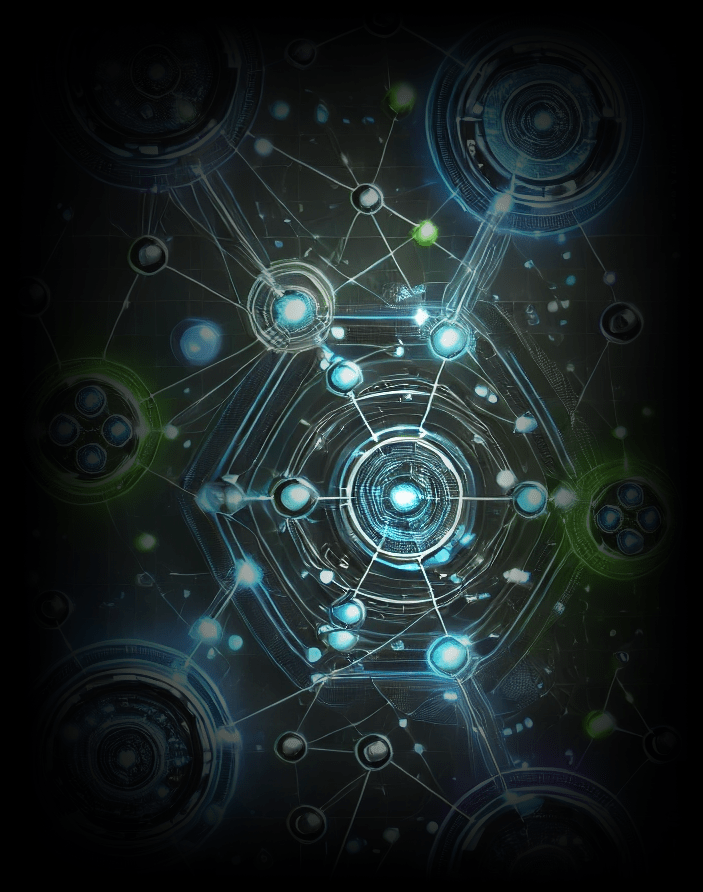
Summary of the most important points
In this article, we have introduced the basic concepts and types of AI agents, including AI Agents, Multi Agents, Sequential Agents and Swarm Agents. We have highlighted the technological foundations, how they work and the diverse areas of application of these agents. By presenting specific example agents, we were able to illustrate the practical implementation and benefits of AI agents.
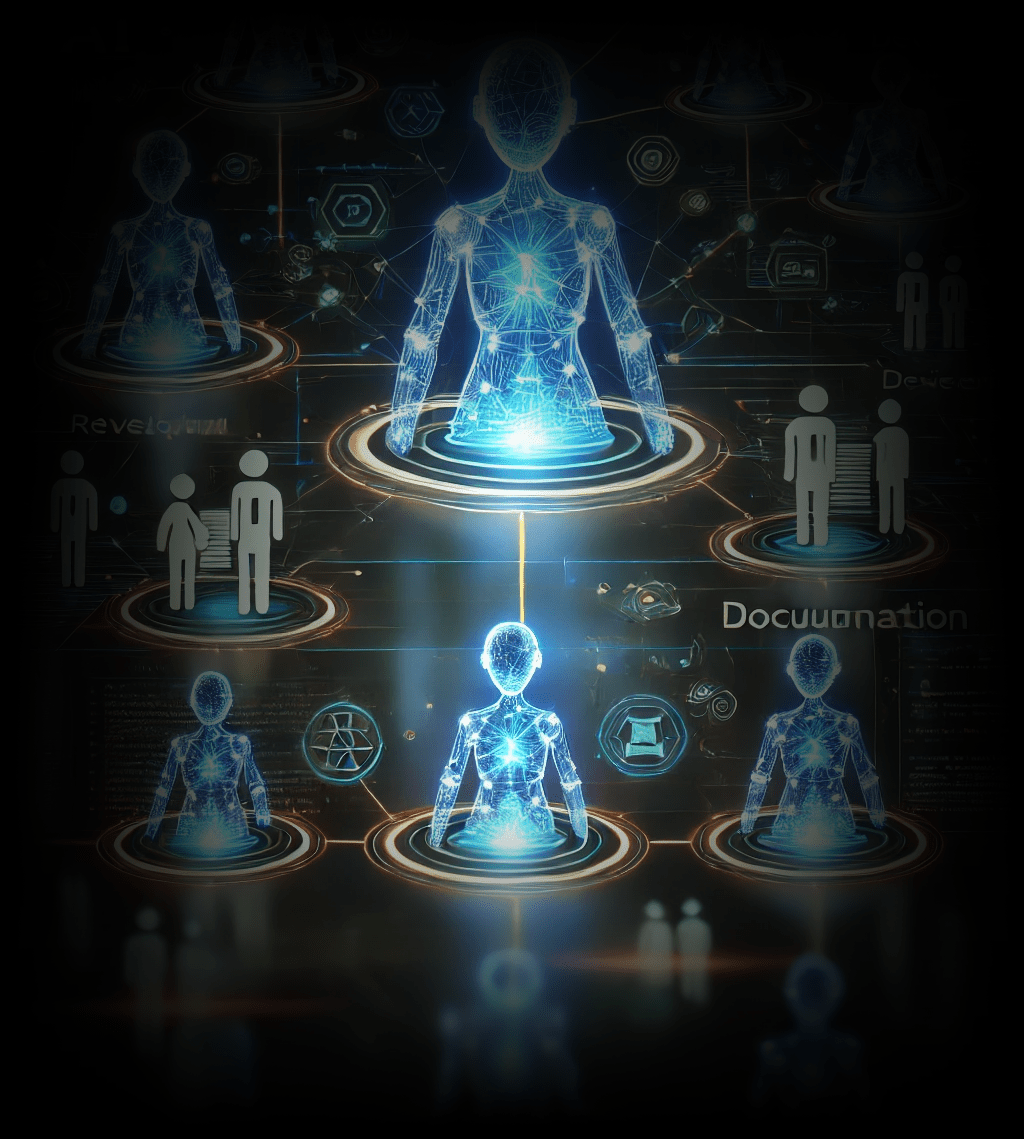
Outlook on the future of AI agents
The development of AI agents is only just beginning, and the future holds many exciting possibilities. As technology advances, AI agents will become even more powerful, autonomous and versatile. The integration of multimodality, improved ethical standards and increasing interdisciplinarity will further expand the possible applications and lead to innovative solutions for global challenges. In particular, the further development of swarm agents will open up new ways to efficiently manage complex, distributed and dynamic tasks.
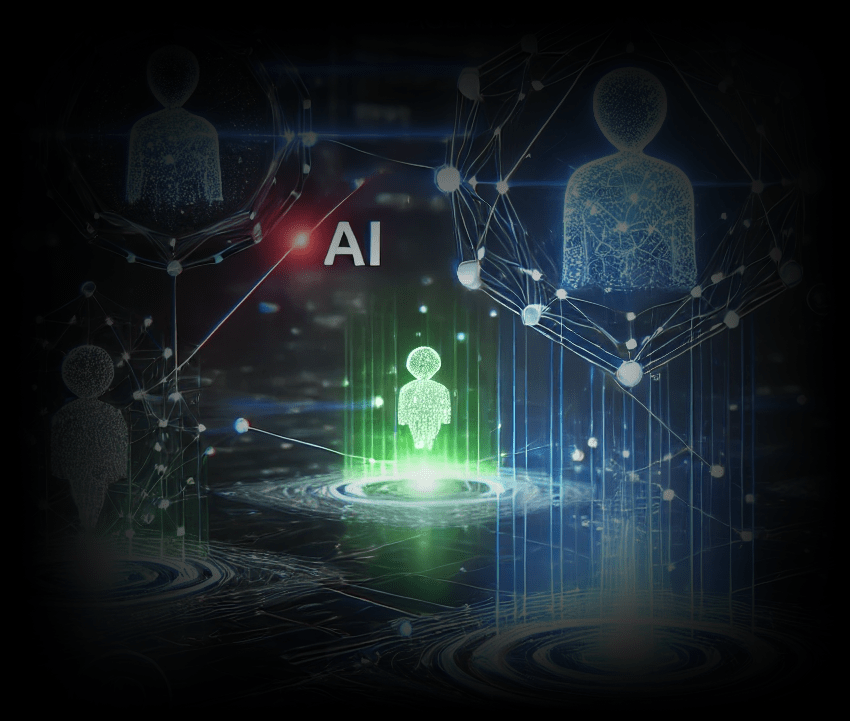
Invitation to interact
We cordially invite you to try out the demo agents presented and experience the many possibilities of AI agents for yourself. Test the functions, ask questions and give us your feedback. Your experiences and suggestions are valuable for continuously improving our AI agents and optimally tailoring them to your needs.
Contact us
Appointment (Google Meet VideoCall) with Chris Wagner
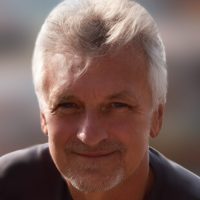
Chris Wagner
More than 30 years of experience
Or request via this form: